Mapping the micro and macro of biology with spatial omics and AI
37 trillion. That is the number or cells that form a human being. How they all work together to sustain life is possibly the biggest unsolved puzzle in biology. A group of up-and-coming technologies for spatially resolved multi omics, here collectively called “spatial omics,” may provide researchers with the solution.
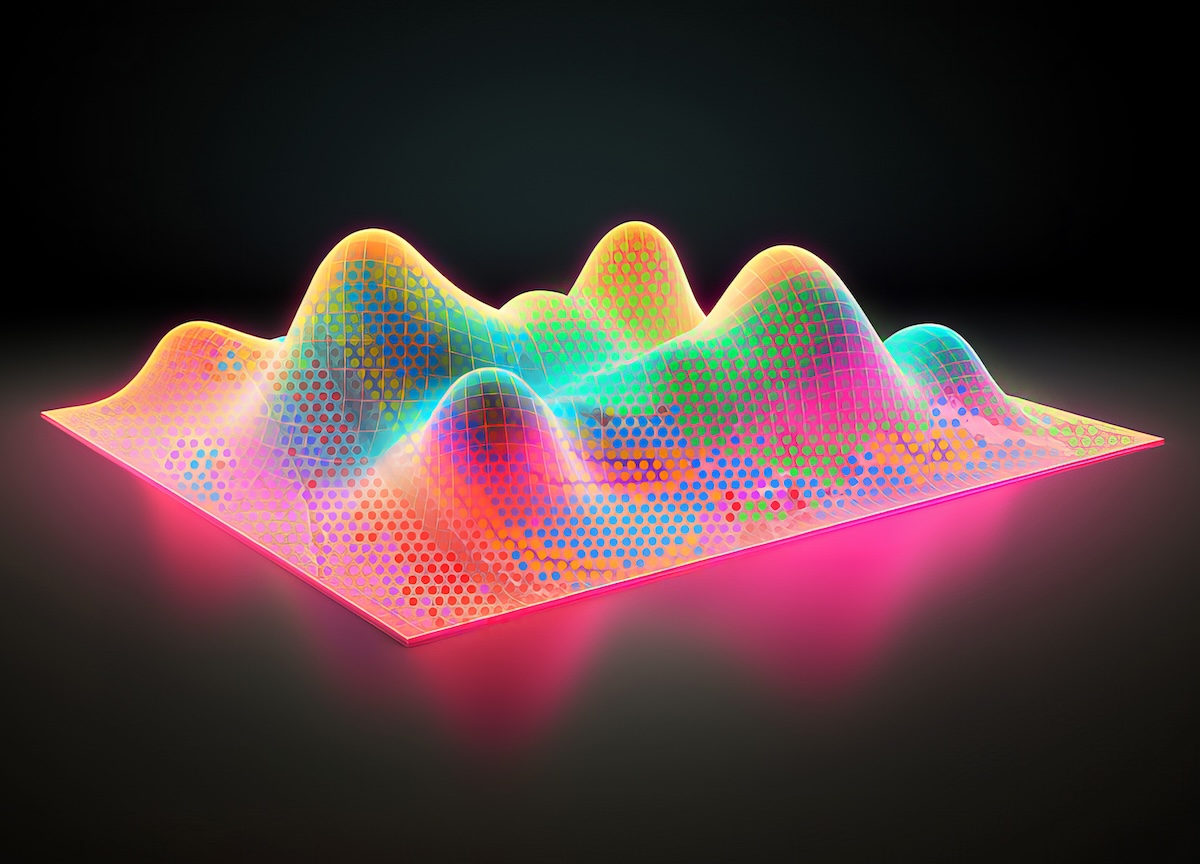
Over the last 20 years, the omics revolution has enabled us to understand cell and tissue biology at ever increasing resolutions. Bulk sequencing techniques that emerged in the mid 2000s allowed the study of mixed populations of cells. A decade later, single-cell omics methods became commercially available, revolutionizing our understanding of cell physiology and pathology. These methods, however, required dissociating cells from their tissue of origin, making it impossible to study their spatial organization in tissue.
Spatial omics refers to the ability to measure the activity of biomolecules (RNA, DNA, proteins, and other omics) in situ—directly from tissue samples. This is important because many biological processes are controlled by highly localized interactions between cells that take place in spatially heterogeneous tissue environments. Spatial omics allows previously unobservable cellular organization and biological events to be viewed in unprecedented detail.
A few years ago, these technologies were just prototypes in a handful of labs around the world. They worked only on frozen tissue and they required impractically large amounts of precious tissue biopsies. But as these challenges have been overcome and the technologies commercialized by life science technology providers, these tools have become available to the wider scientific community. Spatial omics technologies are now improving at a rapid pace, increasing the number of biomolecules that can be profiled from hundreds to tens of thousands, while increasing resolution to single-cell and even subcellular scales.
Complementary advances in data and AI will expand the impact of spatial omics on life sciences and health care—while also raising new questions. How are we going to generate the large datasets that are necessary to make clinically relevant discoveries? What will data scientists see in spatial omics data through the lens of AI?
Discovery requires large-scale spatial omics datasets
Several areas of life science are already benefiting from discoveries made possible by spatial omics, with the biggest impacts in cancer and neurodegenerative disease research. However, spatial omics technologies are very new, and experiments are challenging and costly to execute. Most present studies are performed by single institutions and include only a few dozen patients. Complex cell interactions are highly patient-specific, and they cannot be fully understood from these small cohorts. Researchers need the data to enable hypothesis generation and discovery.
This requires a shift in mentality toward collaborative projects, which can generate large-scale reference datasets both on healthy organs and human diseases. Initiatives such as The Cancer Genome Atlas (TCGA) have transformed our understanding of cancer. Similar large-scale spatial omics efforts are needed to systematically interrogate the role of spatial organization in healthy and diseased tissues; they will generate large datasets to fuel many discovery programs. In addition, collaborative initiatives steer further improvement of spatial omics technologies, generate data standards and infrastructures for data repositories, and drive the development and adoption of computational tools and algorithms.
At Owkin we are pioneering the generation of such datasets. In June 2023, we launched an initiative to create the world largest spatial omics dataset in cancer, with a vision to include data from 7,000 patients with seven difficult-to-treat cancers. The project, known as MOSAIC (Multi-Omics Spatial Atlas in Cancer), won’t stop at the data generation, but will mine the data to learn disease biology and identify new molecular targets against which to design new drugs.
Owkin is well placed to drive this kind of initiative. We can tap a vast network of collaborating hospitals across the globe: to create the MOSAIC dataset, we are working with five world-class cancer research hospitals. And we have deep experience in AI: In the last five years, we have published 54 research papers generating AI methodological innovation and building predictive models in several disease areas, including many types of cancer.
AI’s transformative role in discovering new biology
Spatial omics was recognized as method of the year 2020 by Nature Methods, and it was named one of the top 10 emerging technologies by the World Economic Forum in 2023—alongside generative AI.
With these two technologies developing in tandem, the opportunities for AI-driven biological discoveries from spatial omics are numerous. Looking at the fast-evolving landscape of spatial omics AI methods, we see two broad categories of new methods breaking through.
In the first category are AI methods that aim to improve the usability of spatial omics and enable richer downstream analyses for researchers. Such methods are specially designed to deal with the high dimensionality and the signal-to-noise ratio that are specific to spatial omics. Some are used to remove technical artifacts and batch effects from the data. Other methods, collectively known as “super-resolution methods,” use AI to increase the resolution of spatial omics assays to near single-cell levels. Another group of approaches looks to integrate dissociated single-cell omics with spatial omics. Collectively, these AI methods are bridging the gap with future spatial omics technologies.
In the second category, AI methods aim at discovering new biology from spatial omics. By exploiting the localization information of spatial omics, they shed light on how groups of cells organize and communicate with unprecedented resolution. Such methods are sharpening our understanding of how cells interact to form complex tissues.
At Owkin, we are developing methods to identify new therapeutic targets and patient subpopulations using spatial omics. We have pioneered methods allowing researchers to understand how cancer patient outcomes are linked to tumor heterogeneity, directly from tumor biopsy images. Building on this expertise and the MOSAIC consortium, we are developing the next generation of AI methods, which will link patient-level outcomes with an understanding of disease heterogeneity at the molecular level.
Looking ahead
Spatial biology has the potential to radically change our understanding of biology. It will change how we see a biomarker, going from the mere presence of a particular molecule in a sample to patterns of cells expressing a certain molecule in a tissue. Promising research on spatial biomarkers has been published for several diseases, including Alzheimer’s disease and ovarian cancer. Spatial omics has already been used in research associated with clinical trials to monitor tumor progression in patients.
Five years from now, spatial technologies will be capable of mapping every human protein, RNA, and metabolite at subcellular resolution. The computing infrastructure to store and analyze spatial omics data will be in place, as will the necessary standards for data and metadata and the analytical algorithms. The tumor microenvironment and cellular composition of difficult-to-treat cancers will be mapped through collaborative efforts such as MOSAIC.
Spatial omics datasets from patient biopsies will quickly become an essential part of pharmaceutical R&D, and through the lens of AI methods, they will be used to inform the design of new, more efficacious drugs and to drive faster and better-designed clinical trials to bring those drugs to patients. In the clinic, spatial omics data will routinely be collected from patients, and doctors will use purpose-built AI models to extract clinically relevant information about a patient’s tumor and what drugs it will best respond to.
Today we are witnessing the convergence of three forces: spatial omics technologies becoming increasingly high-throughput and high-resolution, large-scale datasets from patient biopsies being generated, and AI models becoming ever more sophisticated. Together, they will allow researchers to dissect the complex biology of health and diseases, enabling ever more sophisticated therapeutic interventions.
Davide Mantiero, PhD, Joseph Lehár, PhD, and Darius Meadon also contributed to this piece.
This content was produced by Owkin. It was not written by MIT Technology Review’s editorial staff.